KEYNOTE SPEAKERS
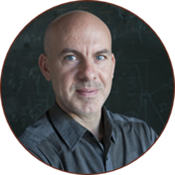
Alex ARENAS
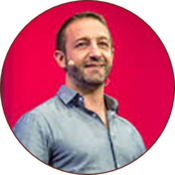
Daniele QUERCIA
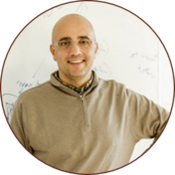
Santo FORTUNATO
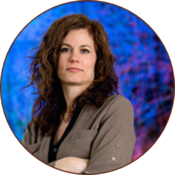
Jennifer NEVILLE
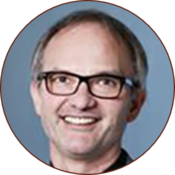
Frank SCHWEITZER
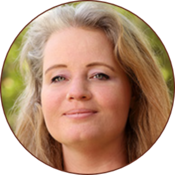
Katharina A. ZWEIG
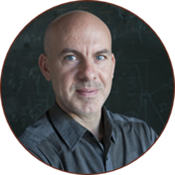
Alex ARENAS
Universitat Rovira i Virgili, Spain
Prof. Alex Arenas (Barcelona, 1969) got his PhD in Physics in 1996. In 1995, he got a tenure position at Dept. Computer Science and Mathematics (DEIM) at Universitat Rovira i Virgili, and in 1997 he became associate professor at the same department. In 2000, he was visiting scholar at the Lawrence Berkeley Lab. (LBL) in the Applied Mathematics group of Prof. Alexandre Chorin (University of California, Berkeley). After this visit, he started a collaboration with Berkeley, and in 2007 he became visiting researcher of LBL. Arenas has written more than 160 interdisciplinary publications in major peer reviewed including Nature, Nature Physics, PNAS, Physics Reports and Physical Review Letters, which have received more than 9000 citations. He is one of the few Europeans serving as Associate Editors of the most important publication in physics worldwide, the American Physical Society journal, Physical Review. He is in charge of the Complex Networks and Interdisciplinary Physics section of Physical Review E. He got the James Mc Donnell Foundation award for the study of complex systems in 2011. He was also recognized as ICREA Academia-Institució Catalana de Recerca i Estudis Avançats, a catalan award that promotes the most recognized scientists from Catalonia. He serves as Editor in Journal of Complex Networks, and in Network Neuroscience. He was elected for the Steering Committee of the Complex Systems Society in 2012. He is the leader of the research group ALEPHSYS.
Finding the most versatile nodes in highly multidimensional data
The determination of the most central agents in complex networks is important
because they are responsible for a faster propagation of information, epidemics,
failures and congestion, among others. A challenging problem is to identify them in
networked systems characterized by different types of interactions, forming
interconnected multilayer networks. Here we describe a mathematical framework
that allows us to calculate centrality in such networks and rank nodes accordingly,
finding the ones that play the most central roles in the cohesion of the whole structure, bridging together different types of relations. These nodes are the most
versatile in the multilayer network. We investigate empirical interconnected
multilayer networks and show that the approaches based on aggregating—or
neglecting—the multilayer structure lead to a wrong identification of the most
versatile nodes, overestimating the importance of more marginal agents and
demonstrating the power of versatility in predicting their role in propagation
processes with applications in social networks, banking networks, etc.
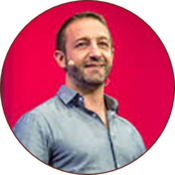
Daniele QUERCIA
NOKIA Bell Labs, UK
Daniele Quercia is a computer scientist and is currentlybuilding the Social Dynamics team at Bell Labs CambridgeUK, has been named one of Fortune magazine's 2014Data All-Stars, and spoke about “happy maps” at TED. Hisresearch area is urban computing. His research receivedbest paper awards from ACM Ubicomp 2014 and fromAAAI ICWSM 2015, and an honorable mention from AAAIICWSM 2013. He was Research Scientist at Yahoo Labs, aHorizon senior researcher at The Computer Laboratory of the University ofCambridge, and Postdoctoral Associate at the Massachusetts Institute ofTechnology. He received his PhD from UC London. His thesis was sponsored byMicrosoft Research Cambridge and was nominated for BCS Best British PhDdissertation in Computer Science. During his PhD, he was MBA Technology Fellow atLondon Business School.
Good City Life
The corporate smart-city rhetoric is about efficiency, predictability, and security.“You’ll get to work on time; no queue when you go shopping, and you are safebecause of CCTV cameras around you”. Well, all these things make a cityacceptable, but they don’t make a city great. We are launching goodcitylife.org - aglobal group of like-minded people who are passionate about building technologieswhose focus is not necessarily to create a smart city but to give a good life to citydwellers. The future of the city is, first and foremost, about people, and thosepeople are increasingly networked. We will see how a creative use of networkgenerated data can tackle hitherto unanswered research questions. Can we rethinkexisting mapping tools? Is it possible to capture smellscapes of entire cities andcelebrate good odors? And soundscapes?
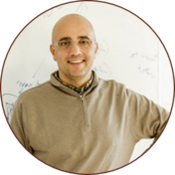
Santo FORTUNATO
Indiana University, USA
He received his PhD in Theoretical Physics in 2000 at theDepartment of Physics of the University of Bielefeld,Germany, working on lattice gauge theories, percolationand phenomenology of heavy-ion collisions. He switchedto complexity science in 2004, and from 2005 till 2007 hehas been postdoctoral researcher at the School ofInformatics and Computing of Indiana University, workingin the group of Alessandro Vespignani. From 2007 till2011 he has been at ISI Foundation in Turin, Italy, first as research scientist then as ascientific leader. In 2011 he became Associate Professor in Complex Systems at theSchool of Science of Aalto University, Finland. He is currently full professor in theSchool of Informatics and Computing at Indiana University.
Community structure in complex networks
Complex systems typically display a modular structure, as modules are easier toassemble than the individual units of the system, and more resilient to failures. Inthe network representation of complex systems, modules, or communities, appearas subgraphs whose nodes have an appreciably larger probability to get connectedto each other than to other nodes of the network. In this talk I will address threefundamental questions: How is community structure generated? How to detect it?How to test the performance of community detection algorithms? I will show thatcommunities emerge naturally in growing network models favoring triadic closure, amechanism necessary to implement for the generation of large classes of systems,like e.g. social networks. I will discuss the limits of the most popular class ofclustering algorithms, those based on the optimization of a global quality function,like modularity maximization. Testing algorithms is probably the single mostimportant issue of network community detection, as it implicitly involves theconcept of community, which is still controversial. I will discuss the importance ofusing realistic benchmark graphs with built-in community structure, as well as therole of metadata.
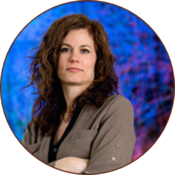
Jennifer NEVILLE
Purdue University, USA
Jennifer Neville is the Miller Family Chair AssociateProfessor of Computer Science and Statistics at PurdueUniversity. She received her PhD from the University ofMassachusetts Amherst in 2006. She is currently anelected member of the AAAI Executive Council and shewas recently PC chair of the 9th ACM InternationalConference on Web Search and Data. In 2012, she wasawarded an NSF Career Award, in 2008 she was chosenby IEEE as one of "AI's 10 to watch", and in 2007 was selected as a member of theDARPA Computer Science Study Group. Her work, which includes more than 100publications with over 5000 citations, focuses on developing data mining andmachine learning techniques for complex relational and network domains, includingsocial, information, and physical networks.
The impact of network structure on relational machine learning
Network science focuses on analyzing network structure in order to understand keyrelational patterns in complex systems. In contrast, relational machine learningtypically conditions on the observed relations in a network, using them as a form ofinductive bias to constrain the space of dependencies considered by the models.While recent interest in these two fields has produced a large body of research onmodels of both network structure and relational data, there has been less attentionon the intersection of the two fields--specifically considering the impact of networkstructure on relational learning methods. Since many relational domains comprise asingle, large, partially-labeled network, many of the conventional assumptions inrelational learning are no longer valid and the network structure creates uniquestatistical challenges for learning and inference algorithms. This talk will outlinesome of the algorithmic and statistical challenges that arise due to partiallyobserved, large-scale networks, and describe methods for semi-supervised learning,latent-variable modeling, and sampling to address the challenges.
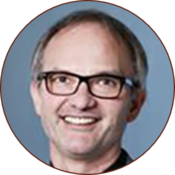
Frank SCHWEITZER
ETH Zurich, Switzerland
Frank Schweitzer has been Full Professor for SystemsDesign at ETH Zurich since 2004. He is also associatedmember of the Department of Physics at the ETH Zurich.Frank Schweitzer received his first Ph.D. (Dr. rer. nat.) intheoretical physics at the age of 26, and his second Ph.D.(Dr. phil.) in philosophy of science at the age of 29, hefurther earned a habilitation/Venia Legendi in Physics. Inhis professional career, he worked for different researchinstitutions (Max-Planck Institute for the Physics of Complex Systems, Dresden,Fraunhofer Institute for Autonomous Intelligent Systems, Sankt Augustin) anduniversities (Humboldt University Berlin, Cornell University Ithaca NY, EmoryUniversity, Atlanta GA). The research of Frank Schweitzer focuses on applications ofcomplex systems theory in the dynamics of social and economic organizations. He isinterested in phenomena as diverse as user interaction in online social networks,collective decisions in animal groups, failure cascades and systemic risk in economicnetworks, and the rise and fall of collaborations in socio-technical systems. Hismethodological approach can be best described as data-driven modeling, i.e., itcombines the insights from big data analysis with the power of agent-basedcomputer simulations and the strength of rigorous mathematical models. FrankSchweitzer is a founding member of the ETH Risk Center and Editor-in-Chief of ACS -Advances in Complex Systems and EPJ Data Science.
Spreading influence in social networks: From link-centric to nodecentric models
Epidemic spreading on complex networks is well studied because nodes follow arather simple dynamic. Thus, the focus is mostly on how the network topologyimpacts the spreading process. However, modeling the spread of, e.g., emotions inonline social networks requires us to have more refined models of the nodedynamics, to calculate cascades of spreading influence. We capture the nodedynamics by means of a data-driven modeling approach that allows us to test, and to calibrate, assumptions about the user behavior. In my talk, I present differentexamples of how to complement the topological perspective by a node-centricperspective that considers costs and benefits, emotional responses or informationprocessing of users.
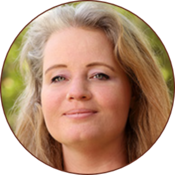
Katharina A. ZWEIG
TU Kaiserslautern, Germany
Katharin A. Zweig is a professor at the TU Kaiserslauternsince 2012. As a studied biochemist and computerscientist, her postdoc was in the biophysics group ofProf. Dr. Tamás Vicsek at ELTE University Hungary. Withthis interdisciplinary background, she designed a newfield of study called Socioinformatics at the TUKaiserslautern. It is concerned with the impact of ITSystems on individuals, organizations, and society atlarge. In her research, Zweig first focused on understanding when to use whichnetwork analytic measure for a meaningful interpretation of the result. Herresearch has now broadened to the meaningful use of other types of data mining.She is a junior fellow of the German Society of Computer Science from 2013 (until2018), was selected as a "Digital Head" in 2014 in Germany, and won the ars-legenditeaching prize in Engineering and Computer Science in 2017. She co-founded aninitiative called "Algorithm Watch" in 2016 and counsels politics, churches, mediaauthorities and foundations with respect to the impact of algorithms on society.
Network analysis literacy: a socioinformatic approach
Why are there so many centrality indices? This is the question that puzzled mewhen I started into network analysis in 2003. Borgatti showed that centrality indicesare best understood as tightly coupled to a specific kind of network flow or networkprocess associated with it. His main idea, that centrality indices come with a modelof a network flow or process, can be generalized to other types of data mining andquality measures. I will thus discuss the question of responsibility when measuresare used in societally important algorithmic decision-making systems, such asterrorist identification systems which include social network features.